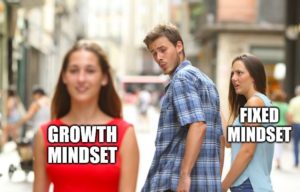
You’ve likely run into the term growth mindset if you’ve read an article or listened to a podcast about learning, success, or child education in recent years. Growth mindset is an idea evangelized by psychologist Carol Dweck throughout decades of research as well as her famous 2007 book Mindset: The New Psychology of Success. Her 2014 TED talk about the idea has accrued over 20 million views across platforms as of early 2023. Dweck and colleagues have repeatedly found that students — and all of us — have differing beliefs about the extent to which intelligence (broadly conceived) is a static, immutable property of a person (she calls this a fixed mindset) or something that can be changed with effort (she calls this a growth mindset).
Based on standardized measures, research finds that some people seem to have more of a fixed mindset and others more of a growth mindset. For example, a student with a fixed mindset might think “I got an F because I’m dumb” or “I’m just not a math person” while one with a growth mindset might think “I got an F this time, so I need to put in more effort and try new strategies” or “math requires a lot of practice, so if I put in the time I could get better at it.”
Basically, those with a growth mindset believe their basic intelligence and abilities can be cultivated through effort and are more likely to persist through setbacks and failures; those with a fixed mindset may shut down or give up when they perform poorly at something.
According to Dweck and colleagues, a growth mindset seems to predict positive outcomes in school (e.g., Claro et al. 2016). Some studies indeed do conclude that students with growth mindset do better in school — or, put another way, students who do well in school appear more likely to have a growth mindset (though that isn’t always true even in the research cited by proponents of the idea).
If it does turn out true that growth mindset predicts performance, it could be that a growth mindset is causing better performance or it actually could be making no difference at all. How is that? Perhaps instead there is some other variable lurking behind the scenes (say, how much parents read to their kids as toddlers) that makes certain students end up higher-performing and also coincidentally makes those same students more likely to have a growth mindset (despite the growth mindset not being what helps them perform).
Does mindset affect performance or merely correlate with it?
Really, this is the usual correlation vs. causation question. So if the correlation between mindset and performance exists, we have to ask whether the growth mindset is actually causing performance improvements or not. The best way to do that is an experimental manipulation. We might try to manipulate mindset (i.e. increase growth mindset) in some people but not others and see if that increases performance in the first group.
But that leads to the meta-question of whether someone’s mindset is even malleable — or are we stuck being a fixed mindset person or a growth mindset person?
Dweck and countless others believe we can inculcate a growth mindset, perhaps using relatively simple and low-cost interventions, and that doing so can improve performance, close achievement gaps (Yeager et al., 2016), and have other benefits. The idea of instilling a growth mindset has become incredibly popular in schools (even though we don’t have any good evidence yet of teacher-based interventions working), but also in the workplace. For example, former CEO of Google Eric Schmidt describes the company’s growth mindset hiring practices.
It’s a powerful idea: that we can get wide-scale improvements in performance and success with some simple training or a change in how we give feedback.
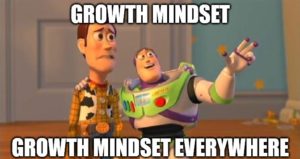
So do mindset interventions actually work? The problem of low quality studies
Whether growth mindset interventions actually work is a surprisingly hard question to answer despite a huge number of research studies aimed at that very question. Diving into the ‘why’ of that challenge can serve as a case study that tells us a lot about the challenges of answering seemingly-simple scientific questions and the temptations of jumping to conclusions based on compelling-sounding storytelling.
In the late 1990s and early 2000s, some (but not all) published studies appeared to show evidence of a mindset intervention working. For example, a classic study compared the effects of praising fifth graders based on their intelligence or based on their effort (Mueller & Dweck, 1998). Those praised for intelligence did worse, showed less persistence, attributed failures to low ability, and cared more about performance goals than genuine learning goals. Other studies found similar patterns, but unfortunately many of these studies were either of low quality or suffered from what we’ve come to call questionable research practices (QRPs).
Questionable research practices are not the same thing as fraud or misconduct, but instead are problematic ways of carrying out a study or analyzing the data that may on the face of it appear fine, but often lead to erroneous conclusions. For example:
- A fishing expedition: if someone collects data on a boat-load of variables (pun intended) and then goes looking for a correlation between every possible combination of all those variables, then they will likely find a few statistical associations in there simply by chance alone. That’s just how statistics work; there’s a small chance of a false positive with each statistical test, so if you run a ton of tests, you have a much higher chance of some false positives. The researcher then might seize on those [false] positive results and shout “eureka!” to the presses about the connections they found between X and Y or between W and Z. But this will be misleading if they don’t tell us that they ran so many statistical tests (and thus there’s a good chance some or all of their results aren’t actually true). A better practice would be to report on all data collected and all analyses run — not just the cherry-picked results — and to correct the statistics to account for all those tests you ran.
- The garden of forking paths: when a researcher has their data in hand, there are often a huge number of possible ways to analyze that data in order to test their hypothesis. Perhaps a researcher uses one method of removing outliers or imputing missing data, or perhaps they try one statistical test that could be used on their data, but then the results come out non-significant and uninteresting (i.e. don’t support their hypothesis). It can be tempting to go back and change the rule you used for outliers or missing data, or to come at the data with a different statistical approach, and then to repeat this until you get significant and interesting results that support your hypothesis. Then only report that final “successful” analysis as how you tested your hypothesis. A better practice would be to publicly pre-register your complete data analysis plan ahead of time and transparently report on any deviations from that plan.
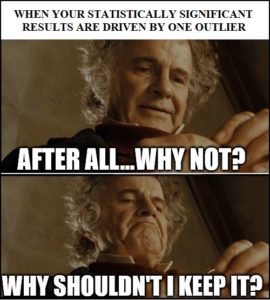
- HARKing (Hypothesizing After Results are Known): normally, one would make a hypothesis ahead of time, then collect and analyze the data to test that hypothesis (and rule out the hypothesis as unlikely if the results don’t fit with it). However, sometimes the researcher’s main hypothesis doesn’t come out statistically significant and the study might seem like a failure, that is, until one thinks up an alternative way of interpreting this unexpected data (a different hypothesis altogether). If the researcher reports their study as if they were testing this other hypothesis all along, then we again increase the risk of false positives (and also can end up solidifying bad theory in the literature). A better practice would be to publicly pre-register your hypothesis and how you plan to test it; then you’re welcome to speculate about alternative interpretations that arose post-hoc as long as you make clear that your new hypothesis came after, not before, seeing the data.
- Too small of a sample: there are equations a researcher can use to calculate how large of a sample (how much data) a researcher needs in order to have a high chance of their statistical test detecting a real effect of some meaningful size (if such an effect exists). For decades, the majority of research in many fields (including psychology) was published based on studies with too small of a sample to have adequate statistical power to detect the effect they were looking for. This, again, leads to myriad false positives and overestimating the size of effects that do happen to be real. A better practice would be to calculate the sample size needed ahead of time and stick to that.
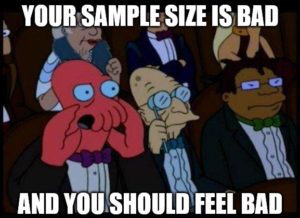
As the statistician Andrew Gelman has pointed out, research by Dweck suffers from problems like the garden of forking paths (i.e. at every step of data collection and analysis, there are so many small decisions in which the researchers can unconsciously bias the outcome by their choices):
[Quote from Haimowitz & Dweck, 2016] “This study included a number of additional measures that are unrelated to the current research question and not reported here.”
Gelman: “Fine, but again, these represent a bunch more lottery tickets that they could’ve tried to cash in, had their first tries not worked.
[Quote from Haimowitz & Dweck, 2016] “There were no effects of child’s age or gender on any of the key variables, so these demographics were not considered in further analyses.”
Gelman: “Points for honesty, but . . . again, forking paths. Interactions with age and sex are two of the paths not taken, that increase the total probability of finding a statistically significant comparison, even if all the responses were noise.” […] “I have no idea whether Haimovitz and Dweck are on to anything here, but it does seem to me that their research designs have enough degrees of freedom that they could take their data to support just about any theory at all.”
Furthermore, mindset proponents seem to change the rules across different studies and it can feel a bit like moving goalposts when looking at how evidence is collected to support their theory. In good science, we should always be looking for evidence that would disconfirm or rule out our hypothesis and that usually means making a clear claim about what that hypothesis predicts and then seeing if that prediction holds true. Yet if you have a vague-enough hypothesis, you can try a near-infinite variety of tests on that hypothesis and then seize onto the tests that happen to confirm your idea while ignoring the ones that don’t. As Jay Lynch points out:
“Each experiment involves wildly disparate choices in how data is collected, analyzed, and measured. Available studies are a dizzying hodgepodge of experiments that are all conceptually related but rely on a growth mindset hypothesis that is so vague and open-ended (e.g., “Growth-mindset interventions improve academic achievement.”) that the researchers are able to claim victory for any observed effect regardless of learning outcome, analyzed subgroup, intervention type, proposed mechanism, or measured impact.
“In one study researchers report the effects of an 8-week malleability training on half-semester math grades, quickly brushing off evidence that the intervention doesn’t seem to help students with a fixed mindset more than those with a growth mindset, and in the next study they are reporting the effects of combining a single 45 minute online growth-mindset course with a completely different sense-of-purpose intervention, and isolating their analysis to at-risk students.”
Beyond that, some of Dweck’s past work has rested on a shaky statistical foundation at the most basic level. In one famous paper (cited more than 1500 times), Dweck and colleagues interpreted and reported what we’d conventionally consider a non-significant result (p-value below 0.10 but above 0.05) as if it was significant (Blackwell et al., 2007).
Likewise, in a more recent study (Haimovitz and Dweck, 2016), she and her colleague made a fundamental and foundational logical error when they claimed that one thing (parents’ attitudes toward failure) has more of an effect than another thing (parents’ attitudes toward intelligence) despite there being no evidence of a difference between those two conditions in their actual data! (They have since issued a correction to that article).
Replications and meta-analyses
Okay, so some of the past research was done in a way that doesn’t inspire great confidence in the results. Aside from improving methodology and avoiding questionable research practices in future work, a couple ways to get a better idea whether an effect is real (or a false positive) are:
- Attempt replications (repeats) of studies that find positive results and see if you find a similar result. Ideally these should be independent replications, meaning they are carried out by researchers not involved with the original results, and ideally they should involve even larger sample sizes and solid research practices.
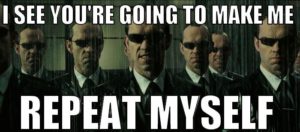
- And do meta-analyses where you analyze the results of many studies (combined) to get an idea of how confident we can be that the effect is real and — if so — a more reliable estimate of how big the effect is. As always when analyzing a bunch of research together, we must beware of the principle Garbage In, Garbage Out, so combining a bunch of low-quality studies still leads to a low-quality meta-analysis; thankfully there are modern techniques to help a meta-analysis weigh results toward higher-quality studies, detect publication bias and other issues, etc.
So what do we find when trying to independently replicate growth mindset interventions? Unfortunately, we get pretty mixed results. Sometimes the interventions seem to work (definitely so when Dweck is involved), but other times these interventions don’t seem to work. One researcher who has tried to replicate Dweck’s work keeps finding null results (i.e. no effect of the intervention); that researcher concludes:
“People with a growth mindset don’t cope any better with failure. If we give them the mindset intervention, it doesn’t make them behave better. Kids with the growth mindset aren’t getting better grades, either before or after our intervention study.” [source]
Meta-analyses (e.g., Sisk et al., 2018) have found weak evidence overall, not just for mindset-changing interventions but for the overall relationship between mindset and achievement. That’s not to say for sure that there isn’t an effect of mindset or that we can’t change mindset, but if so these effects may be small and may only exist in some circumstances or for some subpopulations. For example, Sisk and colleagues conclude that even if interventions don’t help most students, they might still be helpful for at-risk students or those of low socioeconomic status. Another meta-analysis (Burgoyne et al., 2020) again found generally weak (or even contradicting) evidence suggesting that at the very least that the bold claims of mindset interventions have often been overstated.
The huge effect sizes claimed earlier in the mindset literature were hilariously improbable, as Scott Alexander argued back in 2015, and now it looks like we’re dealing with a small and selective effect, if any. That’s not to say small effective sizes aren’t important, if they do in fact exist. Even a small bump, if it applied to millions of people, could be meaningful and worthwhile (depending on cost and trade offs), but we still need to decide if that bump exists.
Dweck has engaged with these criticisms (e.g., Yeager & Dweck, 2020), sometimes picking at the methods of those trying to replicate her work or carry out meta-analyses, but also basically admitting that, yes, the effect seems to be small and only shows up for some people and in some contexts (Hecht et al., 2021), rather than being a broadly-true principle of learning or something that can help anyone do better. (This is a far cry from the TED-talk-esque proselytizing we’ve heard along the way).
While she does thankfully address her critics directly rather than ignoring the issues and questions that have arisen, it’s a little hard to see her defense as objective when she not only makes a ton of money off of her mindset books, but also is reported to charge $20,000 for speaking engagements and also her Brainology program charges $20-50 per student. When there is that much financial gain at stake — in addition to professional clout and fame — it just screams conflict of interest. (She has at least divested from Mindset Works, a company she co-founded).
Meanwhile, even as Dweck tries to mildly temper expectations about mindset interventions in schools (especially as delivered by teachers or parents), schools continue to widely roll out mindset programs.
What can be learned from this case study?
Whether mindset predicts performance and whether we can actually change mindset — those are interesting and important questions (especially given how much time and money is being invested in growth mindset training within schools today). However, this topic can also serve as a case study for more general issues that plague our popular understanding and adoption of scientific claims that make it into the mainstream based on shakier foundations than we realize.
We’ve seen other psychological claims go mainstream only to collapse under scrutiny. For example, Amy Cuddy’s work on “power posing” claimed meaningful real-world effects from simply performing a couple one-minute poses of the body. Her work blew up in the mainstream — indeed, her TED talk has over 68 million views as of early 2023 — yet fell apart under later scientific scrutiny. Power posing doesn’t work as claimed and the original results for it rested on a foundation of questionable research practices. Why do we as a society keep falling into the trap of going wild on a new scientific idea before it’s really been vetted and verified, the work checked and the hype cut through?
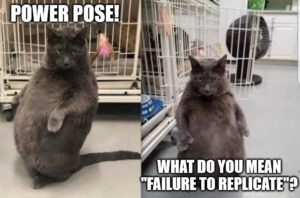
I think Jay Lynch has a good take on this stuff: we like stories that provide surprising and satisfying explanations, and we seize on the initial (often weak) evidence for such explanations as if we’ve discovered a great truth. “Straight to the TED talks!” we say, and society starts adopting this new understanding in schools or workplaces — and let’s not worry about first taking time to confirm whether that initial evidence holds up. We like a nice, tight, simple yet compelling story.
With mindset research, that compelling story was that mindset is a bigger contributor to school success than IQ and that small interventions in mindset can make big differences; the real story is, at best, much messier, much more nuanced, and indeed much more in question than all that hype would lead us to believe. Indeed, the deeper you dive into it, the more it seems to fall apart at a basic theoretical level.
Lynch’s piece nicely walks through the history of mindset research and how easily we can end up with a bunch of studies that seem to lend scientific support to a claim and yet actually provide rather poor evidence for that claim. Does that mean growth mindset doesn’t predict outcomes or that we can’t give people more of a growth mindset?
Not necessarily. Those things could end up being true. In fact, there’s a decent chance they are true, to a much smaller extent than originally claimed and perhaps only for some people. But that’s often how the world works, and how a mature science looks for complex subjects like the human mind.
Effects tend to be heterogenous, that is, the size of the effect differs for different groups and situations (and may even have a non-existent or opposite effect for some). We need to systematically study this heterogeneity, not just stumble upon it when trying to save the grandiose claims of our research after others don’t replicate it or find much smaller effect sizes. Indeed, one of Dweck’s collaborators, David Yeager, and his colleagues have recently pushed this idea in a Perspective article in the journal Nature Human Behavior.
Just remember: next time you’re listening to a TED talk with some compelling story about a big effect scientists have discovered about human psychology, it’s possible those claims came about from research using questionable research practices, and even if the effect genuinely exists, you’re probably hearing some seriously over-hyped claims about it. The truth may end up a little too boring and messy for a TED talk.
And in many cases that catchy, compelling scientific story you’ve heard a million times might turn out completely untrue. For example, did you know the whole idea of learning styles has no real scientific support? That the whole concept is considered thoroughly debunked by psychological scientists? Yet belief in the concept is incredibly widespread and we find near-ubiquitous adoption and praise of learning styles within schools, all based on cherry-picked evidence from some low-quality, shoddy studies. Why? Because the idea that everyone has a unique learning style is a simple, compelling story and we love those.
So even if it turns out mindset doesn’t make a big difference or interventions don’t work, it may be too late to undo the popular misconception.
References
Alexander, S. (2015, April 8). No clarity around growth mindset. Slate Star Codex. https://slatestarcodex.com/2015/04/08/no-clarity-around-growth-mindset-yet/
Andrade, C. (2021). HARKing, cherry-picking, P-hacking, fishing expeditions, and data dredging and mining as questionable research practices. Journal of Clinical Psychiatry, 82(1), 20f13804. https://doi.org/10.4088/JCP.20f13804 [PDF]
Blackwell, L.S., Trzesniewski, K. H., & Dweck, K. S. (2007). Implicit theories of intelligence predict achievement across an adolescent transition: A longitudinal study and an intervention. Child Development, 78(1), 246-263. https://doi.org/10.1111/j.1467-8624.2007.00995.x [SciHub PDF]
Blad, E. (2016, September 20). Teachers seize on ‘growth mindset,’ but crave more training. EducationWeek. https://www.edweek.org/leadership/teachers-seize-on-growth-mindset-but-crave-more-training/2016/09
Bolger, N., Zee, K. S., Rossignac-Milon, M., & Hassin, R. R. (2019). Causal processes in psychology are heterogenous. Journal of Experimental Psychology General, 148(4), 601-618. https://doi.org/10.1037/xge0000558 [PDF]
Bryan, C. J., Tipton, E., & Yeager, D. S. (2021). Behavioural science is unlikely to change the world without a heterogeneity revolution. Nature Human Behaviour, 5, 980-989. https://doi.org/10.1038/s41562-021-01143-3 [SciHub PDF]
Burgoyne, A. P., Hambrick, D. Z., & Mcnamara, B. N. (2020). How firm are the foundations of mind-set theory? The claims appear stronger than the evidence. Psychological Science, 31(3), 258-267. https://doi.org/10.1177/0956797619897588 [SciHub PDF]
Button, K. S., Ioannidis, J. P. A., Mokrysz, C., et al. (2013). Power failure: Why small sample size undermines the reliability of neuroscience. Nature Reviews Neuroscience, 14, 365-376. https://doi.org/10.1038/nrn3475 [PDF]
Chivers, T. (2017, January 14). A mindset ‘revolution’ sweeping Britain’s classrooms may be based on shaky science. BuzzFeed News. https://www.buzzfeed.com/tomchivers/what-is-your-mindset
Claro, S., Paunesku, D., & Dweck, C. S. (2016). Growth mindset tempers the effects of poverty on academic achievement. Proceedings of the National Academy of Sciences, 113(31), 8664-8668. https://doi.org/10.1073/pnas.1608207113 [PDF]
Cuddy, A. (2012). Your body language may shape who you are [Video]. TED. https://www.ted.com/talks/amy_cuddy_your_body_language_may_shape_who_you_are/
Dominus, S. (2017, October 18). When the revolution came for Amy Cuddy. New York Times. https://www.nytimes.com/2017/10/18/magazine/when-the-revolution-came-for-amy-cuddy.html
Dweck, C. S. (2014). The power of believing that you can improve [Video]. TED. https://www.ted.com/talks/carol_dweck_the_power_of_believing_that_you_can_improve
Dweck, C. S. (2017, January 18). Growth mindset is on a firm foundation, but we’re still building the house. Student Experience Research Network. https://studentexperiencenetwork.org/growth-mindset-firm-foundation-still-building-house/
Foliano, F., Rolfe, H., Buzzeo, J., et al. (2019). Changing mindsets: Effectiveness trial. Educational Endowment Foundation. https://discovery.ucl.ac.uk/id/eprint/10118795/
Gelman, A. (2016, May 12). Indeed. I googled *haimowitz and dweck psych science* and found this press release from the Association for Psychological Science, which linked to this paper [Comment on the blog post “Happy talk, meet the Edlin factor”]. Statistical Modeling, Causal Inference, and Social Science. https://statmodeling.stat.columbia.edu/2016/05/12/happy-talk-meet-the-edlin-factor/#comment-272996
Gelman, A. (2018, September 13). Discussion of effects of growth mindset: Let’s not demand unrealistic effect sizes. Statistical Modeling, Causal Inference, and Social Science. https://statmodeling.stat.columbia.edu/2018/09/13/discussion-effects-growth-mindset-lets-not-demand-unrealistic-effect-sizes/
Gelman, A. (2018, October 18). Beyond ‘power pose’: Using replication failures and a better understanding of data collection and analysis to do better science. Statistical Modeling, Causal Infefrence, and Social Science. https://statmodeling.stat.columbia.edu/2017/10/18/beyond-power-pose-using-replication-failures-better-understanding-data-collection-analysis-better-science/
Gelman, A., & Loken, E. (2013). The garden of forking paths: Why multiple comparisons can be a problem, even when there is no ‘fishing expedition’ or ‘p-hacking’ and the research hypothesis was posited ahead of time. Unpublished. http://www.stat.columbia.edu/~gelman/research/unpublished/p_hacking.pdf
Haimovitz, K., & Dweck, C. S. (2016). Parents’ views of failure predict children’s fixed and growth intelligence mind-sets. Psychological Science, 27(6), 859-869. https://doi.org/10.1177/0956797616639727 [SciHub PDF]
Hecht, C. A., Yeager, D. S., Dweck, C. S., & Murphy, M. C. (2021). Beliefs, affordances, and adolescent development: Lessons from a decade of growth mindset interventions. In J. J. Lockman (Ed.), Advances in Child Development and Behavior (pp. 169-197). Academic Press. https://doi.org/10.1016/bs.acdb.2021.04.004 [SciHub PDF]
Lynch, J. (2018, August 17). Growth mindset: The perils of a good research story. Quixotic Scholar. https://medium.com/@quixotic_scholar/growth-mindset-the-perils-of-a-good-research-story-d6ce32a447d2
Khazan, O. (2018, April). The myth of ‘learning styles’. The Atlantic. https://www.theatlantic.com/science/archive/2018/04/the-myth-of-learning-styles/557687/
Meuller, C. M., & Dweck, C. S. (1998). Praise for intelligence can undermine children’s motivation and performance. Journal of Personality and Social Psychology, 75(1), 33-52. https://doi.org/10.1037/0022-3514.75.1.33 [SciHub PDF]
Pashler, H., McDaniel, M., Rohrer, D., & Bjork, R. (2008). Learning styles: Concepts and evidence. Science in the Public Interest, 9(3), 105-119. https://doi.org/10.1111/j.1539-6053.2009.01038.x [PDF]
Sisk, V .F., Burgoyne, A. P., Sun, J., et al. (2018). To what extent and under which circumstances are growth mind-sets important to academic achievement? Two meta-analyses. Psychological Science, 29(4), 549-571. https://doi.org/10.1177/0956797617739704 [SciHub PDF]
Yeager, D. S., & Dweck, C. S. (2020). What can be learned from growth mindset controversies? American Psychologist, 75(9), 1269-1284. https://doi.org/10.1037/amp0000794 [PDF]
Yeager, D. S., Walton, G. M., Brady, S. T., et al. (2016). Teaching a lay theory before college narrows achievement gaps at scale. Proceedings of the National Academy of Sciences, 113(24), E3341-E3348. https://doi.org/10.1073/pnas.1524360113 [PDF]
Leave a Reply